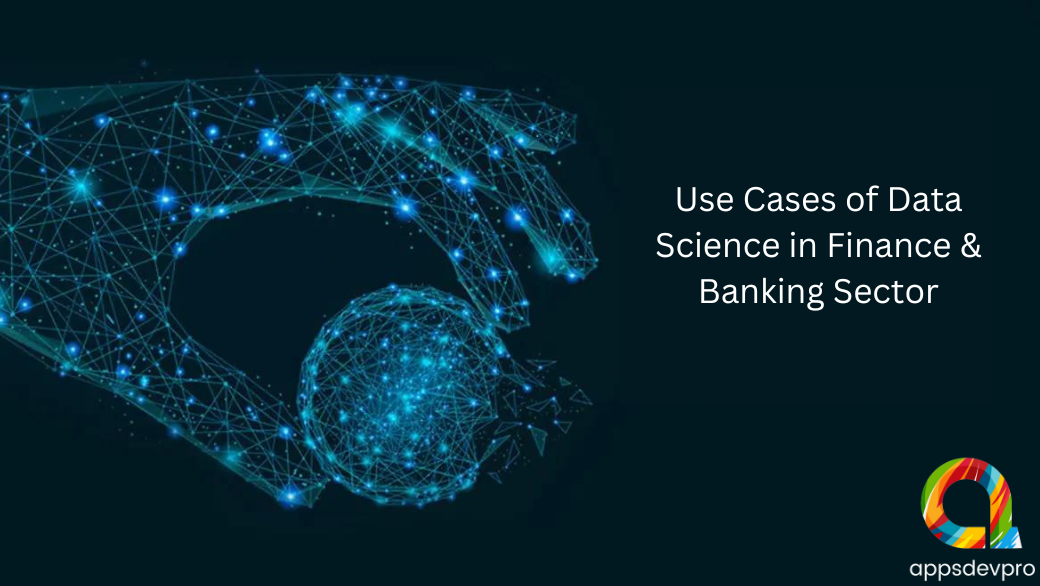
Quick Summary Blog:
Data science in banking and finance is taking over traditional banking solutions and leading to better decision-making, more effective resource allocation, and improved performance. Let’s dig into the details of this blog to understand how data science in the finance industry is roaring and explore 10 use cases of data science in the banking and finance sector.
Start your dream project?
We have a TEAM to get you there.Today, using data science in finance and banking sector has become more than just a trend.
Those are the days past when people were using cheques, or make bank visits for deposits, and using ATMs for withdrawals. In this digital-driven era, everything has become far easier, quicker, and simpler. Digital transformation has made it easy to manage transactions right from mobile apps.
Here’s the infographic stating that the banking and financial sector has the highest adoption rate.
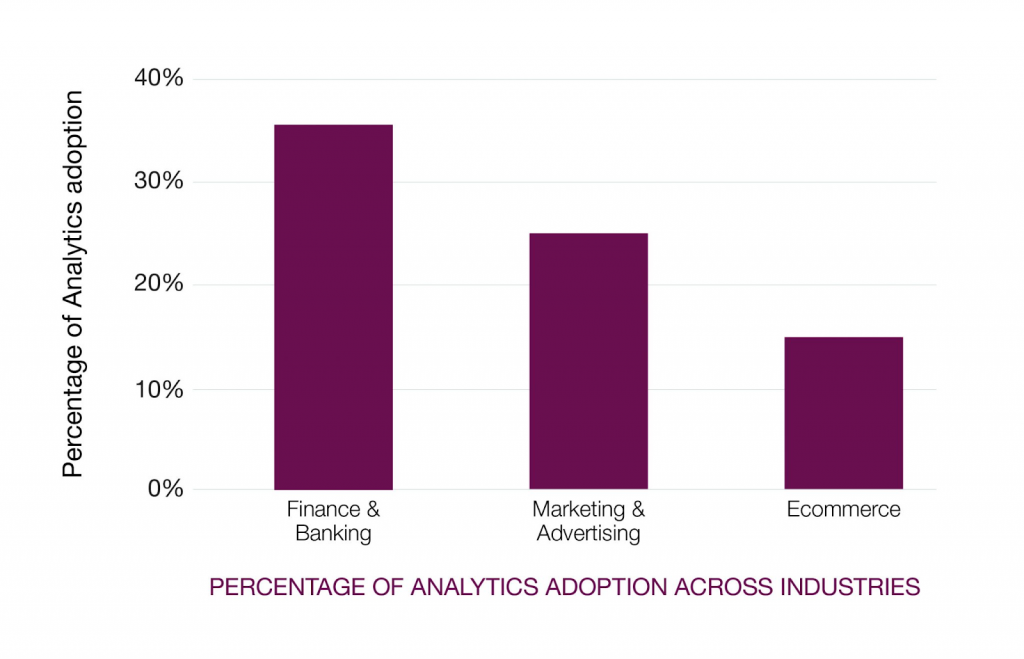
Image Source: pressablecdn.com
However, the major flip slide of increasing digitization in the banking and finance sector is theft, fraud, crimes, and more. That’s where banking institutes have to adopt a solution that ensures data security and maintains customer privacy. And implementing Data science in banking sector is one of the major solutions to all such problems.
If you still rely on traditional banking models, you’re already beyond the market competition. To keep up with the competition, it’s now an alarming time for banks to realize how data science is empowering the banking sector.
Introduction of Data Science in Finance and Banking Sector
The use of data science in finance Industry is revolutionizing the sector. Financial institutions are now utilizing the power of data to gain insight into customers, improve operational efficiency, detect fraud, and make better decisions.
By leveraging data science techniques, banks and other financial organizations are able to better understand their customers, provide more personalized services, reduce risks, and generate greater profits.
Data science has enabled banks and financial services firms to gain a better understanding of customer needs, build customized products, and improve customer experience. It has also enabled them to identify emerging trends in the market, detect potential frauds early on, identify high-risk customers, and evaluate the impact of economic policies.
The possibilities for using data science in banking and finance are endless. Here are a few ways that you can use Data Science in Finance industry by hiring mobile app developers in India:
- Banks can use it to develop predictive models that can help identify potential loan defaulters and detect fraud in real-time.
- They can also use it to build sophisticated algorithms that can improve their portfolio management strategies and optimize their stock investments.
- Also, data science can be used to create stress tests that can help banks assess the stability of their financial positions in a volatile market.
This way, data science is increasingly becoming an integral part of the banking and finance sector. With its ability to generate insights from large amounts of data, banks are able to make smarter and faster decisions that can increase profits and ensure the safety of their customers.
But before you get straight into the process of hiring an app developer in India, let’s understand what exactly is data science and why banks need data science.
What is Data Science?
Data Science is a broad term that encompasses the methods and techniques used to extract insights from large datasets. It is a multidisciplinary field that combines mathematics, computer science, artificial intelligence, statistics, and machine learning to analyze data, uncover patterns, and make predictions.
It is being increasingly used in the banking and finance sector to solve complex problems, develop new products and services, and increase customer satisfaction.
Data Science majorly relies on data analytics, which involves collecting, cleaning, processing, analyzing and interpreting large sets of data. This data can come from different sources such as customer transactions, financial records, surveys, customer reviews, and market trends. The ultimate goal of Data Science is to help businesses make better decisions and improve their operations.
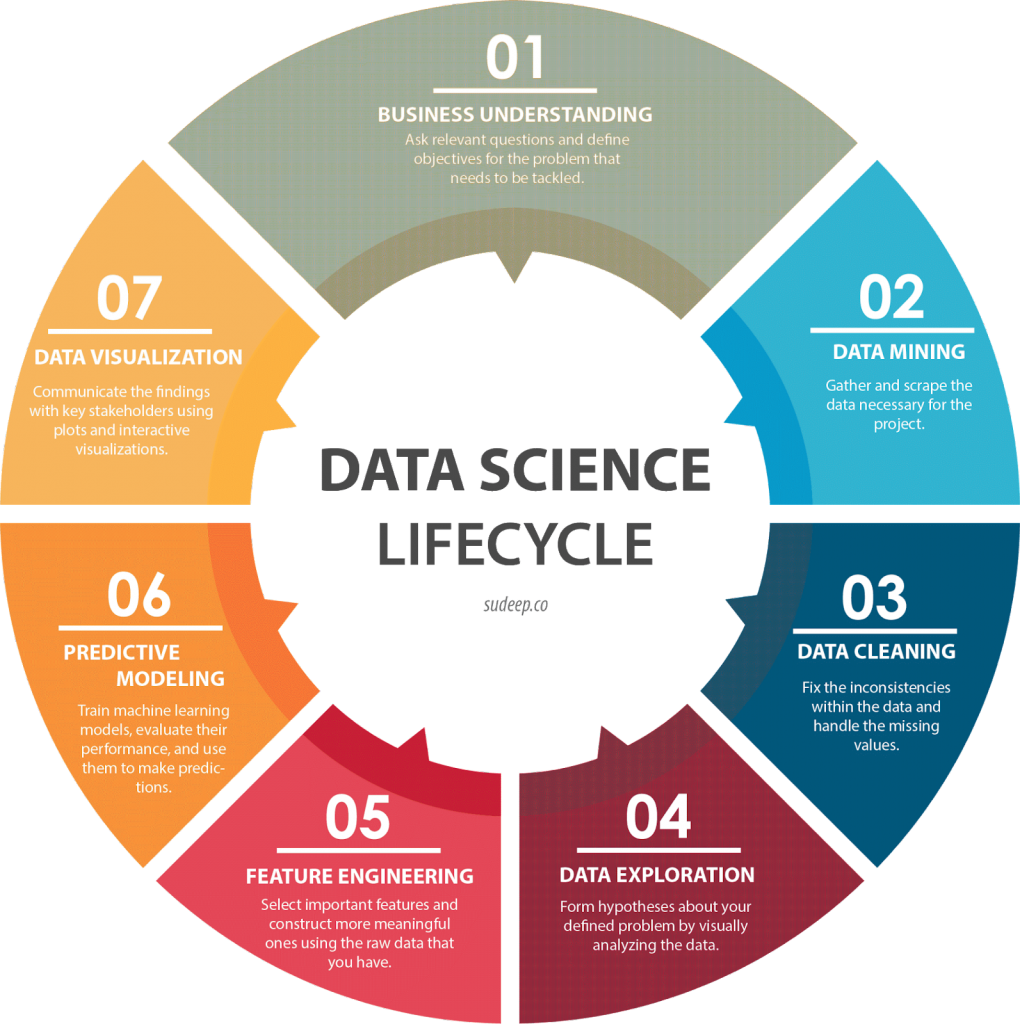
Image Source: educba.com
In the banking and finance sector, Data Science has revolutionized the way banks operate by helping them become more efficient, secure, and profitable. By hiring a software developer in India, banking institutes can improve their financial systems.
The potential applications of Data Science in finance sector are endless. From automated fraud detection to customer segmentation to loan default prediction, Data Science is playing a crucial role in transforming the banking and finance industry.
Let’s look forward to the use cases of data science in finance sector..
Top 11 Use Cases of Data Science in Finance and Banking Sector in 2024
Whether you are already in the banking sector or planning to get started with the banking services, here are a few common ways of using data science in finance industry in 2024.
Use Case 1# Automated Fraud Detection
The increasing use of technology in banking and financial services has made the sector more vulnerable to fraud. Data science is becoming an essential tool for combatting fraud in banking and finance.
According to a report by the Association of Certified Fraud Examiners, losses due to fraud in the banking and financial services industry increased by 17% in 2019.
With the help of data science, banks and other financial institutions are able to detect fraudulent activities in a timely manner and prevent substantial losses.
Data science helps banks detect fraud through the analysis of large datasets. Banks typically use data mining techniques to identify suspicious patterns that are associated with fraudulent activities.
For example, they may look for patterns in customer spending habits or credit card usage that are out of the ordinary. These patterns can be used to flag potentially fraudulent transactions and alert bank staff to investigate further.
In addition, machine learning algorithms can be used to create fraud detection models. These models are trained using historical data and are then used to predict future fraudulent activity. By leveraging such models, banks are able to catch fraudsters before any damage is done.
Data science can also help financial institutions improve their fraud prevention measures. For instance, banks can use natural language processing (NLP) to analyze customer feedback on social media and detect any potentially fraudulent behavior. Banks can then take appropriate steps to ensure that their customers’ accounts remain safe from fraud.
Overall, automated fraud detection through data science is becoming an essential part of the banking and financial services industry. By leveraging data science tools, banks can quickly detect fraudulent activities and protect their customers’ assets.
Looking for top web development services in India to enhance your data science projects in finance? Hire us for expert solutions and seamless integration.
Use Case 2# Customer Segmentation
Customer segmentation is one of the most important and widely used data science techniques in the banking and finance sector. It allows organizations to better understand their customers and identify opportunities to improve customer experience, tailor services, and increase revenue.
Customer segmentation is the process of dividing customers into different groups or clusters based on shared characteristics, such as age, gender, location, spending habits, and more. By understanding customer segments and their needs and preferences, banks can tailor offers and marketing strategies to better reach the right people.
For example, a bank might identify a segment of affluent customers and design an exclusive rewards program to attract them. Alternatively, the bank might target customers with lower incomes with no-fee or low-cost banking services. By using data science to accurately identify different customer segments, banks can create tailored offers that are more likely to be successful.
Customer segmentation also helps banks measure customer engagement and loyalty. By understanding which customers are more engaged and loyal, banks can focus their resources on the most profitable customers. Additionally, segmentation can help banks measure customer lifetime value and develop targeted strategies to retain valuable customers.
Overall, customer segmentation is an invaluable tool for banks to understand their customers and tailor their services accordingly. By leveraging data science to accurately identify customer segments and understand their needs, banks can increase customer satisfaction, loyalty, and revenue.
Use Case 3# Churn Prevention
Churn prevention is an important use case of data science in finance and banking industry. Banks and financial institutions use data science to identify customers who are at risk of leaving the organization.
This is done by analyzing customer behavior data such as frequency of transactions, types of transactions, payment history, etc. With this data, banks can then take proactive measures to prevent customers from leaving by offering them personalized products and services.
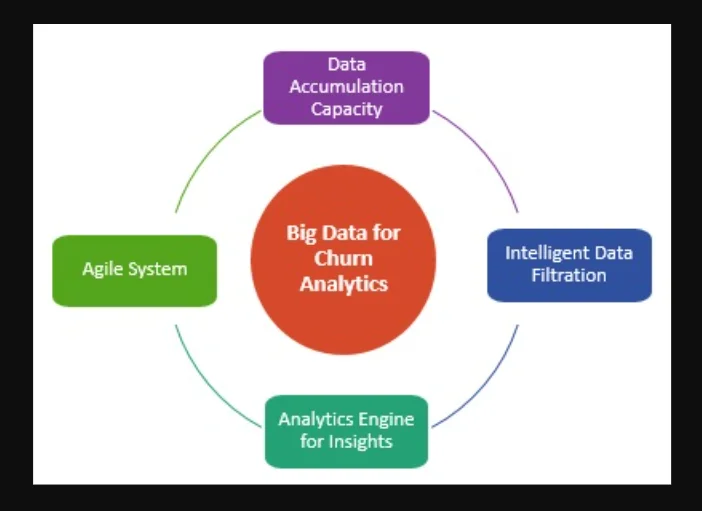
Image Source: tcs.com
All-in-all, a bank might use customer data to determine which customers have the highest likelihood of switching and then offer them a loyalty program or discounts on specific services to retain them. All they need is to hire an India app developer to implement Daya Science in finance industry flawlessly.
Data science can also be used to identify changes in customer spending patterns that could indicate a higher likelihood of churn. By leveraging data science, banks can significantly reduce customer attrition rates and improve customer retention.
Use Case 4# Lifetime Value Prediction
Lifetime value prediction is an important aspect of data science in banking and finance sector. It involves predicting the net present value of a customer over a defined period of time.
The prediction is based on past customer behavior, as well as customer characteristics such as age, gender, location, income level, and spending habits. This is one of the most important uses of data science in finance sector, as it allows banks to optimize their marketing strategies and make more efficient use of their resources.
In order to make accurate lifetime value predictions, data scientists will use a combination of machine learning algorithms, statistical analysis, and predictive modeling techniques. These techniques can analyze large amounts of customer data to accurately predict future customer behavior and their associated revenue.
Benefits of Using Data Science in Finance Industry:
- Helping banks to analyze their customer base,
- Make them understand customer needs and preferences,
- Effectively allocate resources to maximize long-term customer loyalty and profitability,
- Identify high-value customers and offer them personalized rewards,
- Predicting future customer behavior,
- Allowing target their marketing efforts to potential customers and increase their revenues.
Overall, lifetime value prediction provides banks with the insights they need to identify high-value customers and maximize their customer lifetime value. By utilizing data science in banking and finance sector, banks can drive long-term profitability and customer loyalty, leading to increased revenues and customer satisfaction.
Use Case 5# Loan Default Prediction
Loan default prediction is one of the most powerful use cases of data science in finance sector. To make it work smoothly, you can choose to hire Indian app developers that enable you to implement Data Science in banking services.
Now the central question is, how does Data Science work in detecting loan defaults?
By analyzing past loan applications, data scientists can accurately predict the likelihood of a potential borrower defaulting on a loan. This predictive capability helps banks and other financial institutions to manage their credit risk and optimize their lending processes.
Statistics show that the average loss on loan defaults is estimated to be around 5-6% of total loans issued each year.
With data science, banks can reduce these losses by accurately predicting which borrowers are likely to default and taking necessary steps to ensure that the loans are adequately monitored and managed.
Technologies That Help Performing Loan Default Prediction Successfully
Data scientists use advanced machine learning techniques such as logistic regression, support vector machines, neural networks, and other advanced algorithms.
These algorithms use various factors such as the applicant’s credit score, income level, employment history, and other demographic information to create a predictive model. This model is then used to generate scores that indicate the risk of a potential borrower defaulting on their loan.
By using data science to accurately predict loan defaults, banks can reduce the losses associated with defaulting borrowers and improve their lending processes. It also helps them to increase their profitability while providing better services to their customers.
Read more: How to create a money transfer app like Splitwise and its cost.
Use Case 6# Identifying Finance Risks
Financial risks can have a major impact on an institution’s balance sheet, making it vital for organizations to identify and manage them properly. Data science can play a major role in this area by helping banks uncover hidden risks and anticipate future developments.
By leveraging machine learning algorithms, institutions can detect anomalies in customer data or spot correlations between certain variables that may indicate financial risk. Data science also enables banks to develop sophisticated stress testing models that can simulate extreme market scenarios and provide insights into how the bank will perform under different conditions.
Additionally, data science can help banks identify patterns in past financial crises and use them to anticipate future downturns. With the help of data science, banks can detect and manage financial risks more effectively, ensuring their success for years to come.
Use Case 7# Stress Testing
Stress testing is a type of analysis that uses data science to determine the vulnerability of an institution’s financial system. It assesses a bank’s ability to cope with unexpected shocks and financial losses that may arise from economic, operational or other risks.
Stress tests evaluate a bank’s risk management strategies, capital adequacy, liquidity, and overall portfolio strength. To make it work accurately, make sure you hire software developers in India to implement data science in financial services. They also allow regulators to see whether the bank is able to withstand sudden market shocks or changes in its operating environment.
The purpose of stress testing is to identify potential problems before they occur and develop appropriate strategies for mitigating those risks. Banks use stress tests to simulate scenarios such as adverse economic conditions, higher interest rates, or rapid market changes. These simulations help them determine how well their existing resources can handle such situations and what adjustments need to be made if necessary.
By analyzing past events, banks can make informed decisions about future risks and adjust their strategies accordingly. Stress testing allows banks to anticipate and prevent financial losses, as well as build stronger risk management systems.
In addition, stress tests provide valuable insights into a bank’s risk profile, which can be used to inform its decision-making and ensure regulatory compliance.
Use Case #8 Predicting Stock Prices
Stock prices fluctuate daily, making it difficult to make long-term investments or even trade on a short-term basis. Data science can be used to develop predictive models that forecast the future price of a stock, allowing investors to make better decisions and potentially earn higher returns.
Data scientists use various machine learning techniques to generate forecasts and predictions for the movement of a particular stock as mentioned below:
- It includes time series analysis, regression analysis, and neural networks. Time series analysis is used to examine how a stock’s past prices have moved and then predict what its prices will do in the future.
- Regression analysis is used to analyze the relationship between a stock’s past and present prices.
- Neural networks are artificial intelligence algorithms that can learn patterns from past data and then predict future prices.
By leveraging these predictive models, data scientists can identify emerging trends and provide valuable insights into the movement of stock prices. This information can help investors maximize their profits by taking advantage of opportunities when they arise.
Additionally, these predictive models can also be used to identify potential risks associated with particular stocks. By forecasting the possible outcomes of certain stocks, investors can protect their investments and minimize losses.
Overall, data science can be a valuable tool for predicting stock prices and helping investors make informed decisions. By leveraging the power of predictive models, data scientists can provide investors with the insight needed to make profitable trades.
Use Case# 9 Next Best Offers For Customers
One of the most important ways to leverage data science in the banking sector is through the use of next best offer (NBO) strategies. NBOs are a method of personalizing customer interactions, allowing banks to provide the best and most relevant products and services to each individual customer.
By leveraging predictive analytics, banks can identify the most suitable offers for their customers based on their past interactions, financial goals, and current needs. With NBOs, banks can anticipate customer needs and drive greater engagement by presenting them with the offers that are most likely to convert.
Additionally, banks can use NBOs to increase loyalty and satisfaction by delivering tailored experiences that address customers’ unique preferences.
This strategy allows banks to gain a better understanding of customers’ behaviors, as well as identify areas where they could improve their services. Furthermore, NBOs can be used to boost customer acquisition rates and optimize cross-sell/upsell opportunities.
Use Case# 10 Risk Management
Risk management is a critical part of banking, and data science has become increasingly important to ensure it is done effectively. Data science can be used to identify potential risks and help banks develop strategies to minimize their exposure to those risks.
Banks can use data to analyze the creditworthiness of borrowers and identify potential fraud or credit risks. This helps them to determine appropriate levels of risk exposure, as well as identify customers who may pose higher levels of risk. Data science can also be used to develop predictive models that help banks anticipate future risks and plan accordingly.
For example, data-driven models can be used to assess the risk of mortgage defaults and anticipate changing market trends that may affect the bank’s portfolio.
Use Case 11# Automated Customer Support
Automated customer support is an important application of data science in the banking sector. By leveraging data science algorithms and technologies, banks can provide more efficient and responsive customer service. Automated customer support can be used to quickly answer customer inquiries, improve customer satisfaction, and reduce operational costs.
But the central question is how you can automate customer support.
Data science algorithms can be used to build AI-driven chatbots that can understand natural language and provide automated responses to customers’ inquiries. Machine learning algorithms can also be used to predict customer service requests and proactively offer solutions before customers have a chance to contact the bank.
This technology can also help banks by making the customer service process more efficient. For example, AI-driven chatbots can be used to collect information from customers quickly and accurately, which will save time and resources for both the bank and the customer. In addition, predictive analytics can be used to anticipate customer needs and provide personalized responses to customers’ inquiries.
Finally, banks can use data science to detect suspicious transactions and financial crimes, such as money laundering. By leveraging data-driven insights, banks are better equipped to protect themselves from financial losses due to risk.
Read more: Top Mobile App Ideas in 2024
How Much Does It Cost To Integrate Data Science In Banking and Financial Solutions?
The average cost to implement data science in banking and financial solutions can vary widely based on factors like the complexity of the project, the size of the organization, technology requirements, and data infrastructure.
It may range from $10,000 to $20,000, encompassing expenses for skilled professionals, software tools, data storage, infrastructure setup, and ongoing maintenance.
Still, if you are looking to hire full-stack app developers in India, then AppsDevPro can be your trusted partner and help you implement data science in banking solutions.
Conclusion: How Does AppsDevPro Can Help You Implement Data Science in Financial Systems?
At AppsDevPro, we believe in empowering businesses with the power of data science to create a better tomorrow for financial services.
We understand the importance of using data-driven insights to help organizations in the banking and finance sector optimize their operations and develop smarter products and services. We have the experience, technology, and resources to help you implement the data science solutions you need.
By choosing to hire mobile app developers in India, you’ll be assured of the delivery of innovative and reliable analytics-driven solutions. We leverage the latest technologies and advanced data engineering methods to support our clients’ data science initiatives. Our team works closely with clients to build custom solutions that enable them to gain a competitive advantage.
At AppsDevPro, we recognize that data science is essential for success in today’s fast-paced banking and finance industry. Our goal is to help businesses develop a deep understanding of their customers and markets, enabling them to make the most of their data.
If you’re looking for an experienced team to help you implement data science in your financial systems, contact us today!
FAQs
Why Do Banks Need a Data Science?
Banks need to use data science to remain competitive in today’s ever-changing marketplace. By leveraging the power of data, they can make decisions that improve their products and services, enhance customer experience, and reduce costs. To get started, you can choose to hire an Android app developer in India that can understand your needs and can help banks in many ways, such as:
1. Automated fraud detection – Banks can use data science to detect fraudulent activity by looking for patterns and anomalies in customer behavior.
2. Customer segmentation – Data science can help banks to identify key customer segments and create personalized marketing strategies based on customer profiles.
3. Next best offers – Banks can use data science to find opportunities for cross-selling and up-selling, by identifying customer needs and offering relevant products and services.
4. Churn prevention – Data science can help banks to identify customers at risk of leaving so that banks can implement strategies to retain them.
5. Lifetime value prediction – Banks can use data science to predict customer lifetime value, which can be used to design more effective loyalty programs.
6. Risk management – Banks can use data science to identify, measure and manage financial risks more effectively.
7. Algorithmic trading – Banks can use data science to develop algorithms that can automatically execute trades and manage portfolios in the stock market.
8. Portfolio optimization – Data science can help banks to optimize their portfolios and diversify their investments in order to reduce risk and maximize returns.
9. Predicting stock prices – Banks can use data science to analyze market trends and predict stock prices, helping them to make better investment decisions.
10. Detecting financial crimes – Data science can be used to detect patterns of financial fraud and money laundering by examining suspicious activities within a network of transactions.
11. Loan default prediction – Banks can use data science to identify potential loan defaults and adjust the credit risk accordingly.
12. Identifying financial risks – Data science can help banks to analyze the data related to interest rates, inflation, and market trends in order to identify and manage financial risks.
13. Stress testing – Banks can use data science to simulate different economic scenarios in order to assess the impact on their portfolios and investments.
14. Evaluating the impact of economic policy – Data science can be used to evaluate the impact of economic policies on financial markets, helping banks make more informed decisions.
15. Big data analysis – Data science can help banks analyze large amounts of structured and unstructured data in order to extract valuable insights and optimize operations.
These are the major reasons why do financial institutions should hire app developers in India to implement Data science in financial services.
How Data Science in Banking Is Creating New Processes And Financial Models?
Data science is revolutionizing the banking and finance industry. From automated fraud detection to portfolio optimization, the possibilities are virtually endless when it comes to leveraging data science in banking sector. Businesses in this sector are already reaping the benefits of data science, leveraging it to create new processes and financial models that reduce risk and drive success.
Fraud detection is one of the most common use cases for data science in finance sector. By leveraging complex algorithms, financial institutions can easily detect suspicious activity and protect customers from potential losses.
Customer segmentation is another key application, as it allows businesses to customize their product offerings to better match customer needs and interests. Data science can also be used to identify the next best offers and predict customer lifetime values, helping banks optimize sales strategies.
Churn prevention is another important use case for data science in banking and finance. By analyzing customer behavior and past transaction data, companies can identify customers at risk of leaving, and take proactive steps to increase loyalty and retention rates.
Algorithmic trading is also becoming increasingly popular among financial institutions, as it allows them to make fast, informed decisions with minimal human input. Additionally, data science can be used to optimize portfolios, identify financial risks, and predict stock prices.
Data science is also playing a major role in loan default prediction.
By using predictive analytics and machine learning techniques, banks can analyze customer data such as credit history and income level to estimate the probability of default for a loan applicant.
Data science is also being used in stress testing, which helps financial institutions evaluate the impact of economic policy on their balance sheets and strategies.
Lastly, big data analysis is being utilized by banks to uncover insights from large amounts of unstructured customer data.
In conclusion, data science has transformed the banking sector by creating new processes and financial models that reduce risk and drive success. From automated fraud detection to portfolio optimization, financial institutions have access to a plethora of powerful tools that are changing the way they do business.
How Much Does It Cost To Implement Data Science in Finance Services?
The cost of implementing data science in finance services depends on the complexity of the project, the skill sets required to complete it, and the cost of hiring software developers in India. On average, it could range from a few hundred thousand to millions of dollars.
For a small to a medium-sized financial institution, the cost can range from $20,000 to $40,000, including the cost of software, hardware, and personnel. For larger institutions, the cost could increase to upwards of $50,000 or more.
In addition to the costs for initial implementation, there are ongoing expenses for maintaining the system. This includes costs for training personnel and maintenance, as well as upgrades and modifications to existing systems.
Overall, data science has become increasingly essential in the financial sector. Implementing data science in finance services can improve customer experience, optimize operations, and even drive new revenue streams. Despite the cost of initial implementation, data science can deliver immense returns in the long term.
You can also check our other services:
Hire Mobile App Developers in India, Hire Xamarin App Developer in India, Hire iPhone App Developers in India, Hire Android App Developers in India, Hire Flutter App Developer in India, Hire React Native Developers in India, Hire Kotlin Developer in India, Hire Web Developer in India, Hire PHP Developer in India, Hire Laravel Developer in India, Hire Nodejs Developer in India, Hire Microsoft Developer in India, Hire ASP.NET Developer in India, Hire Angular.js Developer in India, Hire React.js Developer in India, Hire E-commerce Developer in India, Hire Magento Developer in India, Hire WordPress Developer, Hire AI Developers in India, Hire Java Developers in India, Hire Python Developers in India, Hire Javascript Developer in India, Hire AR/VR Developers in India, Hire Blockchain Developers in India